Title: Advanced Statistical Techniques in Psychology
In the realm of psychology, advanced statistical techniques play a crucial role in understanding complex phenomena, analyzing data, and deriving meaningful insights. Let's delve into some of these techniques and their applications:
1. Structural Equation Modeling (SEM)
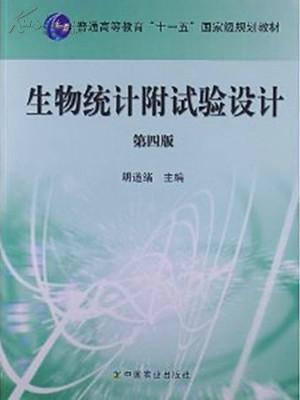
SEM is a powerful statistical method used to test and estimate complex relationships among variables. It allows researchers to examine both direct and indirect effects within a theoretical framework. SEM is commonly used in psychology to assess the validity of theoretical models and understand the interplay between latent constructs.
Application:
Testing causal relationships between latent variables such as personality traits and behaviors.
Evaluating the fit of theoretical models in explaining psychological phenomena.
2. Hierarchical Linear Modeling (HLM)
HLM, also known as multilevel modeling, is used to analyze data that have a hierarchical structure, such as individuals nested within groups. It allows researchers to examine how variables at different levels influence each other.
Application:
Analyzing longitudinal data to understand how individual growth trajectories are influenced by both individual and contextual factors.
Investigating the effects of interventions implemented at different levels (e.g., classroomlevel interventions on student academic performance).
3. Item Response Theory (IRT)
IRT is a framework for analyzing the relationship between latent traits and item responses in psychological assessments, such as personality tests or educational exams. It provides insights into the characteristics of test items and the abilities of testtakers.
Application:
Developing and calibrating psychometric instruments to ensure reliability and validity.
Examining differential item functioning to identify potential biases in assessment tools across different groups.
4. Latent Class Analysis (LCA)
LCA is a statistical method used to identify unobservable subgroups within a population based on patterns of responses to observed variables. It allows researchers to classify individuals into distinct categories based on shared characteristics.
Application:
Identifying subtypes of psychological disorders based on symptom profiles.
Segmenting consumers based on their preferences and behaviors for targeted interventions or marketing strategies.
5. Bayesian Statistics
Bayesian statistics provides a flexible framework for incorporating prior knowledge and updating beliefs based on observed data. It is particularly useful in situations with limited sample sizes or complex models.
Application:
Estimating parameters in psychological models while accounting for uncertainty.
Conducting hypothesis testing and model comparison using Bayesian methods.
Guidance and Recommendations:
1.
Choose the Right Technique
: Selecting the appropriate statistical technique depends on the research question, study design, and characteristics of the data. Consulting with a statistician or experienced researcher can help in making informed decisions.2.
Understand Assumptions
: Familiarize yourself with the underlying assumptions of each statistical method to ensure the validity of the analysis results. Violations of assumptions can lead to biased estimates and incorrect conclusions.3.
Interpret Results Carefully
: Advanced statistical techniques often produce complex output that requires careful interpretation. Consider the practical significance of findings in addition to statistical significance, and communicate results in a clear and understandable manner.In conclusion, advanced statistical techniques play a vital role in advancing our understanding of psychological phenomena. By leveraging these methods effectively, researchers can uncover hidden patterns, test theoretical models, and make meaningful contributions to the field of psychology.